The unprecedented rise of Synthetic Intelligence (AI) has introduced transformative potentialities throughout numerous sectors, from industries and economies to societies at giant. Nonetheless, this technological leap additionally introduces a set of potential challenges. In its current public assembly, the Nationwide AI Advisory Committee (NAIAC)1, which supplies suggestions on subjects together with the present state of the U.S. AI competitiveness, the state of science round AI, and AI workforce points to the President and the Nationwide AI Initiative Workplace, has voted on a discovering based mostly on knowledgeable briefing on the potential dangers of AI and extra particularly generative AI2. This weblog submit goals to make clear these issues and delineate how DataRobot prospects can proactively leverage the platform to mitigate these threats.
Understanding AI’s Potential Dangers
With the swift rise of AI within the realm of know-how, it stands poised to remodel sectors, streamline operations, and amplify human potential. But, these unmatched progressions additionally usher in a myriad of challenges that demand consideration. The “Findings on The Potential Future Dangers of AI” discusses segments the danger of AI in short-term and long-term dangers of AI. The near-term dangers of AI, as described within the discovering, refers to dangers related to AI which might be well-known and present issues for AI, whether or not predictive or generative AI. Then again, long-term dangers of AI underscores the potential dangers of AI that will not materialize given the present state of AI know-how or properly understood however we should always put together for his or her potential impacts. This discovering highlights just a few classes of AI dangers – malicious goal or unintended penalties, financial and societal, and catastrophic.
Societal
Whereas Giant Language Fashions (LLMs) are primarily optimized for textual content prediction duties, their broader functions don’t adhere to a singular purpose. This flexibility permits them to be employed in content material creation for advertising, translation, and even in disseminating misinformation on a big scale. In some cases, even when the AI’s goal is well-defined and tailor-made for a particular goal, unexpected unfavourable outcomes can nonetheless emerge. As well as, as AI techniques evolve in complexity, there’s a rising concern that they may discover methods to bypass the safeguards established to observe or prohibit their habits. That is particularly troubling since, though people create these security mechanisms with explicit targets in thoughts, an AI could understand them in a different way or pinpoint vulnerabilities.
Financial
As AI and automation sweep throughout numerous sectors, they promise each alternatives and challenges for employment. Whereas there’s potential for job enhancement and broader accessibility by leveraging generative AI, there’s additionally a threat of deepening financial disparities. Industries centered round routine actions would possibly face job disruptions, but AI-driven companies may unintentionally widen the financial divide. It’s essential to focus on that being uncovered to AI doesn’t straight equate to job loss, as new job alternatives could emerge and a few staff would possibly see improved efficiency by means of AI help. Nonetheless, with out strategic measures in place—like monitoring labor developments, providing instructional reskilling, and establishing insurance policies like wage insurance coverage—the specter of rising inequality looms, even when productiveness soars. However the implications of this shift aren’t merely monetary. Moral and societal points are taking heart stage. Issues about private privateness, copyright breaches, and our growing reliance on these instruments are extra pronounced than ever.
Catastrophic
The evolving panorama of AI applied sciences has the potential to achieve extra superior ranges. Particularly, with the adoption of generative AI at scale, there’s rising apprehension about their disruptive potential. These disruptions can endanger democracy, pose nationwide safety dangers like cyberattacks or bioweapons, and instigate societal unrest, notably by means of divisive AI-driven mechanisms on platforms like social media. Whereas there’s debate about AI attaining superhuman prowess and the magnitude of those potential dangers, it’s clear that many threats stem from AI’s malicious use, unintentional fallout, or escalating financial and societal issues.
Not too long ago, dialogue on the catastrophic dangers of AI has dominated the conversations on AI threat, particularly as regards to generative AI. Nonetheless, as was put forth by NAIAC, “Arguments about existential threat from AI mustn’t detract from the need of addressing present dangers of AI. Nor ought to arguments about existential threat from AI crowd out the consideration of alternatives that profit society.”3
The DataRobot Method
The DataRobot AI Platform is an open, end-to-end AI lifecycle platform that streamlines/simplifies the way you construct, govern, and function generative and predictive AI. Designed to unify your complete AI panorama, groups and workflows, it empowers you to ship real-world worth out of your AI initiatives, whereas providing you with the flexibleness to evolve, and the enterprise management to scale with confidence.
DataRobot serves as a beacon in navigating these challenges. By championing clear AI fashions by means of automated documentation through the experimentation and in manufacturing, DataRobot allows customers to evaluation and audit the constructing means of AI instruments and its efficiency in manufacturing, which fosters belief and promotes accountable engagement. The platform’s agility ensures that customers can swiftly adapt to the quickly evolving AI panorama. With an emphasis on coaching and useful resource provision, DataRobot ensures customers are well-equipped to grasp and handle the nuances and dangers related to AI. At its core, the platform prioritizes AI security, guaranteeing that accountable AI use isn’t just inspired however integral from improvement to deployment.
Close to generative AI, DataRobot has integrated a reliable AI framework in our platform. The chart beneath highlights the excessive stage view of this framework.
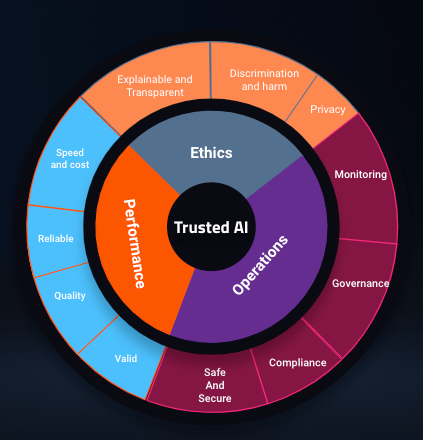
Pillars of this framework, Ethics, Efficiency, and Operations, have guided us to develop and embed options within the platform that help customers in addressing among the dangers related to generative AI. Under we delve deeper into every of those elements.
Ethics
AI Ethics pertains to how an AI system aligns with the values held by each its customers and creators, in addition to the real-world penalties of its operation. Inside this context, DataRobot stands out as an business chief by incorporating numerous options into its platform to handle moral issues throughout three key domains: Explainability, Discrimination and hurt mitigation, and Privateness preservation.
DataRobot straight tackles these issues by providing cutting-edge options that monitor mannequin bias and equity, apply modern prediction rationalization algorithms, and implement a platform structure designed to maximise knowledge safety. Moreover, when orchestrating generative AI workflows, DataRobot goes a step additional by supporting an ensemble of “guard” fashions. These guard fashions play an important position in safeguarding generative use instances. They will carry out duties akin to subject evaluation to make sure that generative fashions keep on subject, determine and mitigate bias, toxicity, and hurt, and detect delicate knowledge patterns and identifiers that shouldn’t be utilized in workflows.
What’s notably noteworthy is that these guard fashions may be seamlessly built-in into DataRobot’s modeling pipelines, offering an additional layer of safety round Language Mannequin (LLM) workflows. This stage of safety instills confidence in customers and stakeholders relating to the deployment of AI techniques. Moreover, DataRobot’s sturdy governance capabilities allow steady monitoring, governance, and updates for these guard fashions over time by means of an automatic workflow. This ensures that moral issues stay on the forefront of AI system operations, aligning with the values of all stakeholders concerned.
Efficiency
AI Efficiency pertains to evaluating how successfully a mannequin accomplishes its meant purpose. Within the context of an LLM, this might contain duties akin to responding to person queries, summarizing or retrieving key info, translating textual content, or avarious different use-cases. It’s value noting that many present LLM deployments typically lack real-time evaluation of validity, high quality, reliability, and value. DataRobot, nonetheless, has the potential to observe and measure efficiency throughout all of those domains.
DataRobot’s distinctive mix of generative and predictive AI empowers customers to create supervised fashions able to assessing the correctness of LLMs based mostly on person suggestions. This ends in the institution of an LLM correctness rating, enabling the analysis of response effectiveness. Each LLM output is assigned a correctness rating, providing customers insights into the arrogance stage of the LLM and permitting for ongoing monitoring by means of the DataRobot LLM Operations (LLMOps) dashboard. By leveraging domain-specific fashions for efficiency evaluation, organizations could make knowledgeable selections based mostly on exact info.
DataRobot’s LLMOps provides complete monitoring choices inside its dashboard, together with velocity and value monitoring. Efficiency metrics akin to response and execution instances are constantly monitored to make sure well timed dealing with of person queries. Moreover, the platform helps the usage of customized metrics, enabling customers to tailor their efficiency evaluations. For example, customers can outline their very own metrics or make use of established measures like Flesch reading-ease to gauge the standard of LLM responses to inquiries. This performance facilitates the continued evaluation and enchancment of LLM high quality over time.
Operations
AI Operations focuses on guaranteeing ith the reliability of the system or the atmosphere housing the AI know-how. This encompasses not solely the reliability of the core system but in addition the governance, oversight, upkeep, and utilization of that system, all with the overarching purpose of guaranteeing environment friendly, efficient, and secure and safe operations.
With over 1 million AI tasks operationalized and delivering over 1 trillion predictions, the DataRobot platform has established itself as a strong enterprise basis able to supporting and monitoring a various array of AI use instances. The platform boasts built-in governance options that streamline improvement and upkeep processes. Customers profit from customized environments that facilitate the deployment of data bases with pre-installed dependencies, expediting improvement lifecycles. Essential information base deployment actions are logged meticulously to make sure that key occasions are captured and saved for reference. DataRobot seamlessly integrates with model management, selling finest practices by means of steady integration/steady deployment (CI/CD) and code upkeep. Approval workflows may be orchestrated to make sure that LLM techniques endure correct approval processes earlier than reaching manufacturing. Moreover, notification insurance policies hold customers knowledgeable about key deployment-related actions.
Safety and security are paramount issues. DataRobot employs two-factor authentication and entry management mechanisms to make sure that solely licensed builders and customers can make the most of LLMs.
DataRobot’s LLMOps monitoring extends throughout numerous dimensions. Service well being metrics monitor the system’s capability to reply rapidly and reliably to prediction requests. Essential metrics like response time present important insights into the LLM’s capability to handle person queries promptly. Moreover, DataRobot’s customizable metrics functionality empowers customers to outline and monitor their very own metrics, guaranteeing efficient operations. These metrics may embody total price, readability, person approval of responses, or any user-defined standards. DataRobot’s textual content drift characteristic allows customers to observe modifications in enter queries over time, permitting organizations to research question modifications for insights and intervene in the event that they deviate from the meant use case. As organizational wants evolve, this textual content drift functionality serves as a set off for brand spanking new improvement actions.
DataRobot’s LLM-agnostic strategy provides customers the flexibleness to pick out essentially the most appropriate LLM based mostly on their privateness necessities and knowledge seize insurance policies. This accommodates companions, which implement enterprise privateness, in addition to privately hosted LLMs the place knowledge seize shouldn’t be a priority and is managed by the LLM house owners. Moreover, it facilitates options the place community egress may be managed. Given the various vary of functions for generative AI, operational necessities could necessitate numerous LLMs for various environments and duties. Thus, an LLM-agnostic framework and operations are important.
It’s value highlighting that DataRobot is dedicated to repeatedly enhancing its platform by incorporating extra accountable AI options into the AI lifecycle for the advantage of finish customers.
Conclusion
Whereas AI is a beacon of potential and transformative advantages, it’s important to stay cognizant of the accompanying dangers. Platforms like DataRobot are pivotal in guaranteeing that the ability of AI is harnessed responsibly, driving real-world worth, whereas proactively addressing challenges.
1 The White Home. n.d. “Nationwide AI Advisory Committee.” AI.Gov. https://ai.gov/naiac/.
2 “FINDINGS: The Potential Future Dangers of AI.” October 2023. Nationwide Synthetic Intelligence Advisory Committee (NAIAC). https://ai.gov/wp-content/uploads/2023/11/Findings_The-Potential-Future-Dangers-of-AI.pdf.
3 “STATEMENT: On AI and Existential Danger.” October 2023. Nationwide Synthetic Intelligence Advisory Committee (NAIAC). https://ai.gov/wp-content/uploads/2023/11/Statement_On-AI-and-Existential-Danger.pdf.
Concerning the creator
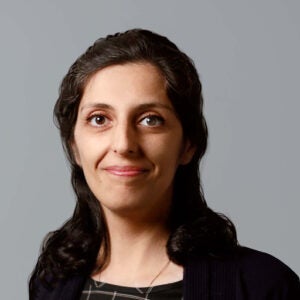
Haniyeh is a World AI Ethicist on the DataRobot Trusted AI workforce and a member of the Nationwide AI Advisory Committee (NAIAC). Her analysis focuses on bias, privateness, robustness and stability, and ethics in AI and Machine Studying. She has a demonstrated historical past of implementing ML and AI in a wide range of industries and initiated the incorporation of bias and equity characteristic into DataRobot product. She is a thought chief within the space of AI bias and moral AI. Haniyeh holds a PhD in Astronomy and Astrophysics from the Rheinische Friedrich-Wilhelms-Universität Bonn.