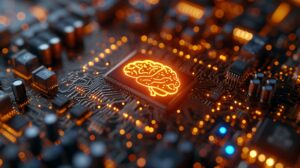
(Treecha/Shutterstock)
Monte Carlo has made a reputation for itself within the discipline of information observability, the place it makes use of machine studying and different statistical strategies to establish high quality and reliability points hiding in large information. With this week’s replace, which it made throughout its IMPACT 2024 occasion, the corporate is adopting generative AI to assist it take its information observability capabilities to a brand new degree.
Relating to information observability, or any sort of IT observability self-discipline for that matter, there isn’t a magic bullet (or ML mannequin) that may detect all the potential methods information can go unhealthy. There’s a big universe of attainable ways in which issues can go sideways, and engineers have to have some thought what they’re on the lookout for to be able to construct the foundations that automate information observability processes.
That’s the place the brand new GenAI Monitor Suggestions that Monte Carlo introduced yesterday could make a distinction. In a nutshell, the corporate is utilizing a big language mannequin (LLM) to go looking by the myriad ways in which information is utilized in a buyer’s database, after which recommending some particular displays, or information high quality guidelines, to keep watch over them.
Right here’s the way it works: Within the Knowledge Profiler part of the Monte Carlo platform, pattern information is fed into the LLM to investigate how the database is used, particularly the relationships between the database columns. The LLM makes use of this pattern, in addition to different metadata, to construct a contextual understanding of precise database utilization.
Whereas classical ML fashions do effectively with detecting anomalies in information, equivalent to desk freshness and quantity points, LLMs excel at detecting patterns within the information which might be troublesome if not inconceivable to find utilizing conventional ML, says Lior Gavish, Monte Carlo co-founder and CTO.
“GenAI’s energy lies in semantic understanding,” Gavish tells BigDATAwire. “For instance, it will possibly analyze SQL question patterns to know how fields are literally utilized in manufacturing, and establish logical relationships between fields (like guaranteeing a ‘start_date’ is all the time sooner than an ‘end_date). This semantic comprehension functionality goes past what was attainable with conventional ML/DL approaches.”
The brand new functionality will make it simpler for technical and non-technical workers to construct information high quality guidelines. Monte Carlo used the instance of an information analyst for knowledgeable baseball workforce to shortly create guidelines for a “pitch_history” desk. There’s clearly a relationship between the column “pitch_type” (fastball, curveball, and so forth.) and pitch velocity. With GenAI baked in, Monte Carlo can mechanically suggest information high quality guidelines that make sense primarily based on the historical past of the connection between these two columns, i.e. “fastball” ought to have pitch speeds of higher than 80mph, the corporate says.
As Monte Carlo’s instance reveals, there are intricate relationships buried in information that conventional ML fashions would have a tough time teasing out. By leaning on the human-like comprehension abilities of an LLM, Monte Carlo can begin to dip into these hard-to-find information relationships to search out acceptable ranges of information values, which is the true profit that this brings.
In response to Gavish, Monte Carlo is utilizing Anthropic Claude 3.5 Sonnet/Haiku mannequin working in AWS. To reduce hallucinations, the corporate carried out a hybrid method the place LLM solutions are validated in opposition to precise sampled information earlier than being offered to customers, he says. The service is absolutely configurable, he says, and customers can flip it off in the event that they like.
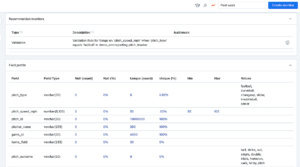
Monte Carlo is utilizing an LLM to mechanically establish relationships between information fields that people would instantly decide up on, equivalent to pitch sort and velocity (Picture courtesy Monte Carlo)
Because of its human-like functionality to understand semantic which means and generate correct responses, GenAI tech has the potential to remodel many information administration duties which might be extremely reliant on human notion, together with information high quality administration and observability. Nevertheless, it hasn’t all the time been clear precisely the way it will all come collectively. Monte Carlo has talked previously about how its information observability software program may also help be certain that GenAI purposes, together with the retrieval-augmented technology (RAG) workflows, are fed with high-quality information. With this week’s announcement, the corporate has proven that GenAI can play a task within the information observability course of itself.
“We noticed a possibility to mix an actual buyer want with new and thrilling generative AI expertise, to supply a means for them to shortly construct, deploy, and operationalize information high quality guidelines that may finally bolster the reliability of their most essential information and AI merchandise,” Monte Carlo CEO and Co-founder Barr Moses stated in a press launch.
Monte Carlo made a few different enhancements to its information observability platform throughout its IMACT 2024 Knowledge Observability Summit, which it held this week. For starters, it launched a brand new Knowledge Operations Dashboard designed to assist prospects observe their information high quality initiatives. In response to Gavish, the brand new dashboard offers a centralized view into numerous information observability from a single pane of glass.
“Knowledge Operations Dashboard offers information groups scannable information about the place incidents are occurring, how lengthy they’re persisting, and the way effectively incidents house owners are doing at managing the incidents in their very own purview,” Gavish says. “Leveraging the dashboard permits information leaders to do issues like establish incident hotspots, lapses in course of adoption, areas inside the workforce the place incident administration requirements aren’t being met, and different areas of operational enchancment.”
Monte Carlo additionally bolstered its help for main cloud platforms, together with Microsoft Azure Knowledge Manufacturing facility, Informatica, and Databricks Workflows. Whereas the corporate may detect points with information pipelines working in these (and different) cloud platforms earlier than, it now has full visibility into pipeline failures, lineage and pipeline efficiency working on these distributors’ methods, Gavish says, together with
“These information pipelines, and the integrations between them, can fail leading to a cascading deluge of information high quality points,” he tells us. “Knowledge engineers get overwhelmed by alerts throughout a number of instruments, battle to affiliate pipelines with the info tables they influence, and haven’t any visibility into how pipeline failures create information anomalies. With Monte Carlo’s end-to-end information observability platform, information groups can now get full visibility into how every Azure Knowledge Manufacturing facility, Informatica or Databricks Workflows job interacts with downstream property equivalent to tables, dashboards, and experiences.”
Associated Gadgets:
Monte Carlo Detects Knowledge-Breaking Code Adjustments