Are you seeing tangible outcomes out of your funding in generative AI — or is it beginning to really feel like an costly experiment?
For a lot of AI leaders and engineers, it’s exhausting to show enterprise worth, regardless of all their exhausting work. In a latest Omdia survey of over 5,000+ international enterprise IT practitioners, solely 13% of have totally adopted GenAI applied sciences.
To cite Deloitte’s latest examine, “The perennial query is: Why is that this so exhausting?”
The reply is complicated — however vendor lock-in, messy knowledge infrastructure, and deserted previous investments are the highest culprits. Deloitte discovered that no less than one in three AI packages fail on account of knowledge challenges.
In case your GenAI fashions are sitting unused (or underused), likelihood is it hasn’t been efficiently built-in into your tech stack. This makes GenAI, for many manufacturers, really feel extra like an exacerbation of the identical challenges they noticed with predictive AI than an answer.
Any given GenAI venture comprises a hefty combine of various variations, languages, fashions, and vector databases. And everyone knows that cobbling collectively 17 completely different AI instruments and hoping for one of the best creates a scorching mess infrastructure. It’s complicated, sluggish, exhausting to make use of, and dangerous to manipulate.
With no unified intelligence layer sitting on high of your core infrastructure, you’ll create larger issues than those you’re attempting to unravel, even if you happen to’re utilizing a hyperscaler.
That’s why I wrote this text, and that’s why myself and Brent Hinks mentioned this in-depth throughout a latest webinar.
Right here, I break down six techniques that can assist you to shift the main focus from half-hearted prototyping to real-world worth from GenAI.
6 Techniques That Substitute Infrastructure Woes With GenAI Worth
Incorporating generative AI into your present methods isn’t simply an infrastructure drawback; it’s a enterprise technique drawback—one which separates unrealized or damaged prototypes from sustainable GenAI outcomes.
However if you happen to’ve taken the time to put money into a unified intelligence layer, you may keep away from pointless challenges and work with confidence. Most corporations will stumble upon no less than a handful of the obstacles detailed beneath. Listed here are my suggestions on how you can flip these widespread pitfalls into progress accelerators:
1. Keep Versatile by Avoiding Vendor Lock-In
Many corporations that wish to enhance GenAI integration throughout their tech ecosystem find yourself in certainly one of two buckets:
- They get locked right into a relationship with a hyperscaler or single vendor
- They haphazardly cobble collectively varied element items like vector databases, embedding fashions, orchestration instruments, and extra.
Given how briskly generative AI is altering, you don’t wish to find yourself locked into both of those conditions. You have to retain your optionality so you may rapidly adapt because the tech wants of your online business evolve or because the tech market modifications. My suggestion? Use a versatile API system.
DataRobot can assist you combine with all the main gamers, sure, however what’s even higher is how we’ve constructed our platform to be agnostic about your present tech and slot in the place you want us to. Our versatile API supplies the performance and suppleness it is advisable to truly unify your GenAI efforts throughout the prevailing tech ecosystem you’ve constructed.
2. Construct Integration-Agnostic Fashions
In the identical vein as avoiding vendor lock-in, don’t construct AI fashions that solely combine with a single software. As an illustration, let’s say you construct an software for Slack, however now you need it to work with Gmail. You may need to rebuild all the factor.
As an alternative, purpose to construct fashions that may combine with a number of completely different platforms, so that you will be versatile for future use circumstances. This gained’t simply prevent upfront growth time. Platform-agnostic fashions will even decrease your required upkeep time, because of fewer customized integrations that have to be managed.
With the appropriate intelligence layer in place, you may deliver the ability of GenAI fashions to a various mix of apps and their customers. This allows you to maximize the investments you’ve made throughout your total ecosystem. As well as, you’ll additionally be capable of deploy and handle a whole bunch of GenAI fashions from one location.
For instance, DataRobot may combine GenAI fashions that work easily throughout enterprise apps like Slack, Tableau, Salesforce, and Microsoft Groups.
3. Convey Generative And Predictive AI into One Unified Expertise
Many corporations wrestle with generative AI chaos as a result of their generative and predictive fashions are scattered and siloed. For seamless integration, you want your AI fashions in a single repository, regardless of who constructed them or the place they’re hosted.
DataRobot is ideal for this; a lot of our product’s worth lies in our capacity to unify AI intelligence throughout a company, particularly in partnership with hyperscalers. Should you’ve constructed most of your AI frameworks with a hyperscaler, we’re simply the layer you want on high so as to add rigor and specificity to your initiatives’ governance, monitoring, and observability.
And this isn’t only for generative or predictive fashions, however fashions constructed by anybody on any platform will be introduced in for governance and operation proper in DataRobot.
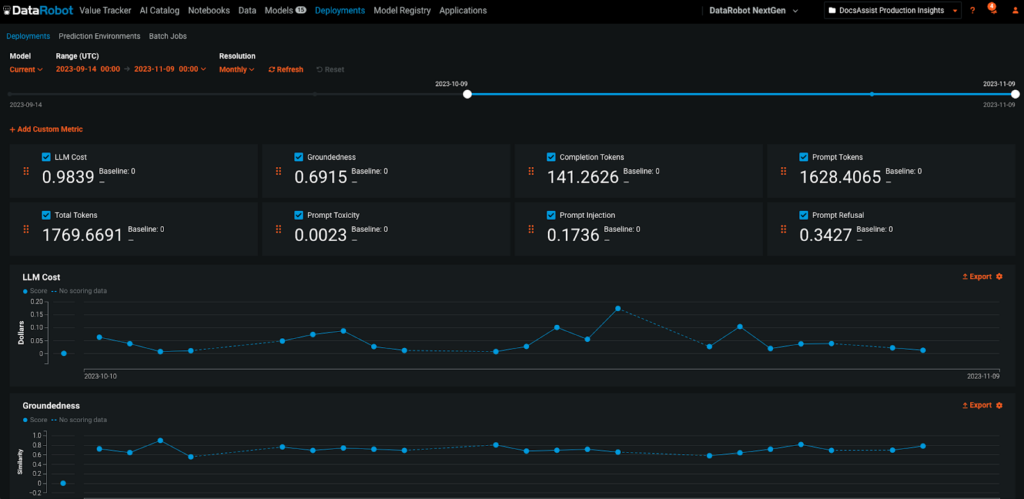
4. Construct for Ease of Monitoring and Retraining
Given the tempo of innovation with generative AI over the previous 12 months, lots of the fashions I constructed six months in the past are already outdated. However to maintain my fashions related, I prioritize retraining, and never only for predictive AI fashions. GenAI can go stale, too, if the supply paperwork or grounding knowledge are outdated.
Think about you have got dozens of GenAI fashions in manufacturing. They could possibly be deployed to every kind of locations comparable to Slack, customer-facing functions, or inner platforms. Ultimately your mannequin will want a refresh. Should you solely have 1-2 fashions, it might not be an enormous concern now, but when you have already got a list, it’ll take you a number of guide time to scale the deployment updates.
Updates that don’t occur by way of scalable orchestration are stalling outcomes due to infrastructure complexity. That is particularly vital once you begin pondering a 12 months or extra down the highway since GenAI updates often require extra upkeep than predictive AI.
DataRobot presents mannequin model management with built-in testing to verify a deployment will work with new platform variations that launch sooner or later. If an integration fails, you get an alert to inform you concerning the failure instantly. It additionally flags if a brand new dataset has extra options that aren’t the identical as those in your presently deployed mannequin. This empowers engineers and builders to be way more proactive about fixing issues, somewhat than discovering out a month (or additional) down the road that an integration is damaged.
Along with mannequin management, I exploit DataRobot to watch metrics like knowledge drift and groundedness to maintain infrastructure prices in test. The straightforward reality is that if budgets are exceeded, tasks get shut down. This could rapidly snowball right into a scenario the place complete teamsare affected as a result of they will’t management prices. DataRobot permits me to trace metrics which are related to every use case, so I can keep knowledgeable on the enterprise KPIs that matter.
5. Keep Aligned With Enterprise Management And Your Finish Customers
The largest mistake that I see AI practitioners make shouldn’t be speaking to folks across the enterprise sufficient. You have to usher in stakeholders early and speak to them usually. This isn’t about having one dialog to ask enterprise management in the event that they’d be all for a selected GenAI use case. You have to repeatedly affirm they nonetheless want the use case — and that no matter you’re engaged on nonetheless meets their evolving wants.
There are three parts right here:
- Have interaction Your AI Customers
It’s essential to safe buy-in out of your end-users, not simply management. Earlier than you begin to construct a brand new mannequin, speak to your potential end-users and gauge their curiosity degree. They’re the buyer, and they should purchase into what you’re creating, or it gained’t get used. Trace: Ensure no matter GenAI fashions you construct want to simply connect with the processes, options, and knowledge infrastructures customers are already in.
Since your end-users are those who’ll finally determine whether or not to behave on the output out of your mannequin, it is advisable to guarantee they belief what you’ve constructed. Earlier than or as a part of the rollout, speak to them about what you’ve constructed, the way it works, and most significantly, the way it will assist them accomplish their targets.
- Contain Your Enterprise Stakeholders In The Improvement Course of
Even after you’ve confirmed preliminary curiosity from management and end-users, it’s by no means a good suggestion to simply head off after which come again months later with a completed product. Your stakeholders will nearly definitely have a number of questions and prompt modifications. Be collaborative and construct time for suggestions into your tasks. This helps you construct an software that solves their want and helps them belief that it really works how they need.
- Articulate Exactly What You’re Attempting To Obtain
It’s not sufficient to have a aim like, “We wish to combine X platform with Y platform.” I’ve seen too many shoppers get hung up on short-term targets like these as an alternative of taking a step again to consider total targets. DataRobot supplies sufficient flexibility that we could possibly develop a simplified total structure somewhat than fixating on a single level of integration. You have to be particular: “We would like this Gen AI mannequin that was inbuilt DataRobot to pair with predictive AI and knowledge from Salesforce. And the outcomes have to be pushed into this object on this means.”
That means, you may all agree on the tip aim, and simply outline and measure the success of the venture.
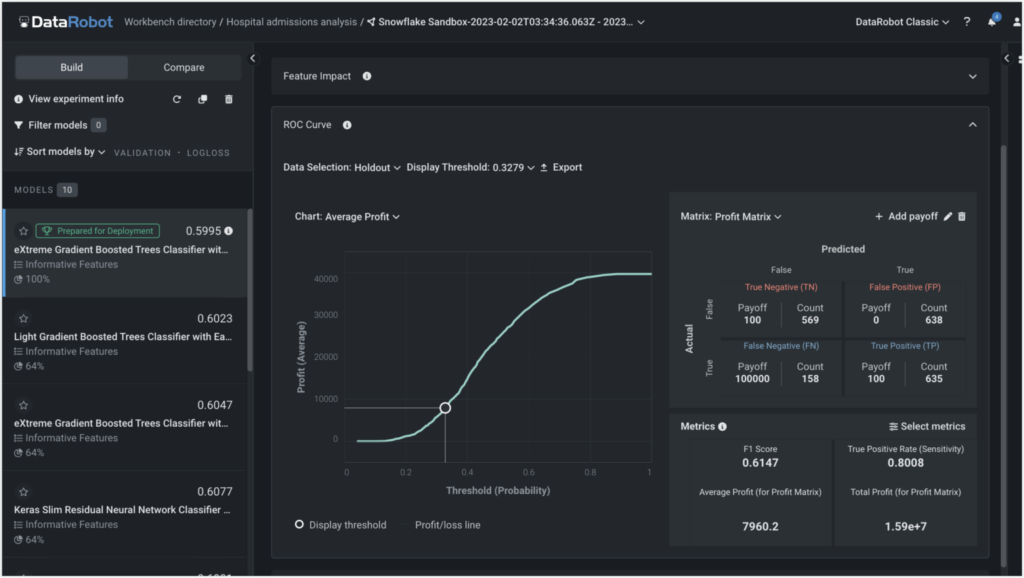
6. Transfer Past Experimentation To Generate Worth Early
Groups can spend weeks constructing and deploying GenAI fashions, but when the method shouldn’t be organized, all the typical governance and infrastructure challenges will hamper time-to-value.
There’s no worth within the experiment itself—the mannequin must generate outcomes (internally or externally). In any other case, it’s simply been a “enjoyable venture” that’s not producing ROI for the enterprise. That’s till it’s deployed.
DataRobot can assist you operationalize fashions 83% sooner, whereas saving 80% of the traditional prices required. Our Playgrounds characteristic provides your workforce the inventive house to match LLM blueprints and decide one of the best match.
As an alternative of constructing end-users anticipate a last answer, or letting the competitors get a head begin, begin with a minimal viable product (MVP).
Get a fundamental mannequin into the palms of your finish customers and clarify that this can be a work in progress. Invite them to check, tinker, and experiment, then ask them for suggestions.
An MVP presents two important advantages:
- You may affirm that you simply’re transferring in the appropriate path with what you’re constructing.
- Your finish customers get worth out of your generative AI efforts rapidly.
When you could not present a excellent person expertise together with your work-in-progress integration, you’ll discover that your end-users will settle for a little bit of friction within the brief time period to expertise the long-term worth.
Unlock Seamless Generative AI Integration with DataRobot
Should you’re struggling to combine GenAI into your present tech ecosystem, DataRobot is the answer you want. As an alternative of a jumble of siloed instruments and AI property, our AI platform may offer you a unified AI panorama and prevent some severe technical debt and trouble sooner or later. With DataRobot, you may combine your AI instruments together with your present tech investments, and select from best-of-breed parts. We’re right here that can assist you:
- Keep away from vendor lock-in and forestall AI asset sprawl
- Construct integration-agnostic GenAI fashions that can stand the take a look at of time
- Preserve your AI fashions and integrations updated with alerts and model management
- Mix your generative and predictive AI fashions constructed by anybody, on any platform, to see actual enterprise worth
Able to get extra out of your AI with much less friction? Get began at this time with a free 30-day trial or arrange a demo with certainly one of our AI consultants.